Introduction: The Privacy-Prediction Paradox
Today, data is both a boon and a challenge. With the right insights, retailers can predict trends, anticipate customer needs, and make every shopping experience feel tailored and seamless. Yet, as powerful as predictive analytics in retail has become, it also raises a key question: is customer data collection intrusive?
Take, for instance, the recent public outcry over excessive data collection by popular brands. Consumers, growing more informed and cautious, are no longer content with vague promises about privacy. They want to know how their data is being used, and they expect transparency and accountability from the brands they support.
Herein lies the paradox: predictive analytics is becoming essential for retailers to thrive in a competitive market, but it’s precisely this dependence on data that threatens to erode the customer trust it aims to foster.
Can retailers find a middle ground? In this blog, we’ll delve into the delicate balance between delivering personalised, predictive experiences and respecting customer privacy. By the end, we hope to answer one pressing question: can retail have it both ways?
Why Predictive Analytics is Essential in Retail
Predictive analytics has become a cornerstone of modern retail, where data-driven insights can make or break a brand. At its core, predictive analytics in retail leverages historical data—such as purchase history, browsing patterns, and seasonal trends—to anticipate customer needs and optimise operations. For example, retailers can offer personalised product recommendations that align with each shopper’s tastes, boosting engagement and loyalty. In inventory management, predictive models allow retailers to stock up on high-demand items and avoid over-ordering, leading to cost savings and better customer satisfaction. Meanwhile, trend forecasting enables brands to stay ahead by recognising shifts in customer preferences, so they’re always ready to meet emerging demands.
For customers, this means a shopping experience that feels relevant and personalised, from well-timed offers to products that match their unique style. For retailers, the benefits are substantial: reduced operational costs, improved customer retention, and increased revenue. In a world of endless online options, these advantages aren’t just perks—they’re essential.
With e-commerce giants constantly raising the bar on convenience and personalisation, brick-and-mortar and hybrid retailers now face immense pressure to keep up. Predictive analytics in retail empowers them to do just that, turning raw data into actionable insights and helping to bridge the gap between physical and digital retail experiences. As competition intensifies, this ability to blend personalisation with efficiency has shifted from being a “nice-to-have” to an indispensable tool in retail survival.
The Privacy Challenge
To power predictive analytics, retailers often rely on a wealth of customer data—purchase history, browsing behaviour, location, and even demographic details. While this data is invaluable for creating personalised experiences, many consumers are increasingly wary about what’s being collected and why.
Some common concerns stem from issues like over-targeting, where customers receive overly precise or intrusive marketing messages. Excessive data collection, where companies gather more information than needed, and a lack of transparency around data usage further amplify these anxieties. When consumers feel tracked or scrutinised, trust in the brand can quickly erode.
As a result, attitudes toward data sharing are shifting. More customers are using ad blockers, opting out of tracking, or even bypassing brands they perceive as invasive. For retailers, this growing resistance to data sharing poses a real challenge, as predictive models rely on consistent and comprehensive data to stay effective. Balancing the benefits of data-driven personalisation with a transparent, privacy-first approach has become critical in building lasting customer relationships.
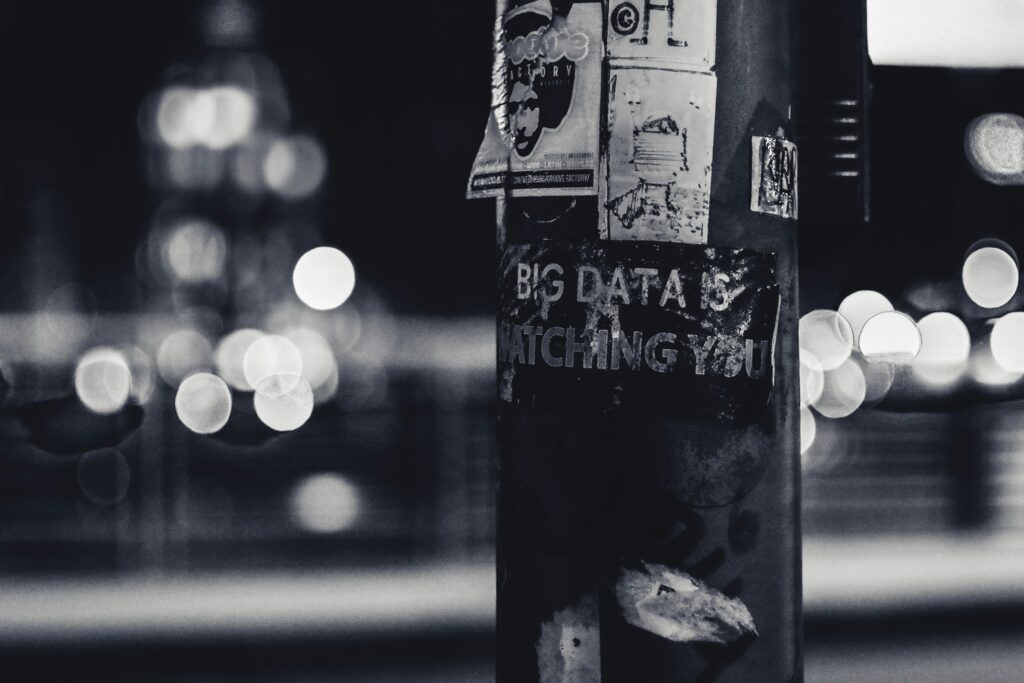
Finding the Balance: Privacy-Respecting Approaches to Predictive Analytics in Retail
Retailers are increasingly adopting privacy-conscious strategies that allow them to harness the power of predictive analytics without compromising customer trust. Data minimisation is one of the most effective approaches, where companies limit data collection to only what’s necessary for insights. By focusing on anonymised and aggregate data, retailers can still extract valuable patterns, like seasonal trends or popular products, without needing personal identifiers. This ensures predictive accuracy while minimising individual customer data usage.
Transparency and consent are also crucial for building trust. By providing clear, accessible privacy policies and allowing customers to opt into data sharing, retailers give shoppers control over their data. Through tiered personalisation options, customers can choose whether they want fully personalised recommendations, general offers, or none at all, ensuring they’re comfortable with their level of data exposure.
Solutions like refive make data capturing less intrusive and more convenient by offering digital receipts that naturally integrate data collection and consent mechanisms. With refive, customers can receive digital receipts and have the option to provide their consent for personalisation, directly from their smartphones. This convenient approach allows retailers to capture opt-ins at the point of sale, enhancing both customer experience and data privacy.
Advanced techniques like differential privacy and data masking further enable retailers to preserve individual privacy while still generating useful insights. Differential privacy adds statistical “noise” to datasets, making it nearly impossible to identify specific individuals while still allowing meaningful trend analysis. Data masking, similarly, replaces sensitive information with anonymised identifiers, enabling retailers to analyse customer behaviours without accessing personally identifiable information. Together, these techniques show that predictive analytics and privacy can indeed coexist, allowing retailers to build personalised experiences that are both effective and respectful.
Customer trust is also being strengthened through data empowerment initiatives. Retailers that educate customers on how their data is used, and the benefits of sharing it responsibly, stand to build stronger, trust-based relationships. By creating transparent campaigns that explain how data-driven personalisation works, customers feel informed and more in control, enhancing both trust and engagement.
Finally, embracing a privacy-first approach provides a competitive edge in the retail market. As consumers increasingly seek out brands that respect their data, companies that prioritise privacy can attract a more loyal, values-driven customer base. This approach not only fosters loyalty but can also enhance brand reputation, positioning retailers as trustworthy, customer-centric, and forward-thinking. Retailers who see privacy as a strategic advantage rather than a hurdle are better placed to thrive in an era where privacy and personalisation go hand in hand.